AC Smart Energy Systems
We develop AI-based solutions that enable the Energiewende and the digitization of power systems
Motivation
To achieve the climate goals and a sustainable energy supply, the traditional, fossil fuel-based, centrally organized supply is increasingly being replaced by volatile renewable sources such as wind and solar. Simultaneously, a decentralized structure of the energy system is emerging, making it possible to use decentrally generated energy as locally as possible, e.g., in the neighborhood, community, or city, without unnecessary transmission. These developments open up new opportunities for the energy system transformation and pose new challenges, such as ensuring a reliable supply in a completely emission-free future.
Goals
At the DAI Laboratory, we investigate how digitization and artificial intelligence can counteract these new challenges posed by the energy transition. Digitization is emerging as a necessary enabler. It creates faster and more flexible market and system processes. Digitization can facilitate the coupling of the power grid to other sectors such as heat supply, industry, and mobility. It enables integrating the end consumer as a “prosumer” – simultaneously as a consumer and producer of energy. But also methods from the field of AI enable to make better decisions within the energy system. Intelligent algorithms can quickly find approximate solutions even in complex situations when traditional methods, such as network simulations, reach their limits due to the high complexity.
Technology
The AC Smart Energy Systems brings together the competence centers’ activities at the DAI Laboratory for future energy systems solutions. For example, machine learning can produce better forecasts (see CC AIM), and complex optimization algorithms can then be used to increase energy efficiency, reduce CO2 emissions or cut costs. In multi-agent systems (see CC ACT), the system’s components are represented by autonomous, intelligent services. These services find optimal strategies through cooperation and independent negotiation without central control. Robotics can also be applied in the energy system (see CC RAS). Drones can independently scan overhead lines for damage, or electric vehicles can be automatically connected to the charging points to serve the grid as energy storage service. The CC SAS is researching how interaction with systems can adapt to the user. We show how the user and the algorithms can interact better together, for example, by visualizing energy and network data through augmented reality to support installers in their work. Cross-cutting technologies like IT security (CC SEC) and reliable communication systems (CC FCS) are important pillars of future reliable energy systems.
Teaching
A great challenge to achieve the digitization and wide-spread utilization of data-driven methods in the energy system is interdisciplinarity. To make use of AI opportunities in the energy system, actors from different backgrounds must work together. This requires interdisciplinary education and training on the one hand, and breaking silo thinking to bring the right actors together on the other. At the DAI-Lab, we supervise interdisciplinary Bachelor and Master theses and offer the project-based course Smart Energy Systems. The course explores the technical, economic, environmental, and policy aspects of microgrids with renewable energy resources, energy storage, and electric vehicles. If interested in (co-)supervision of your thesis at the AC Smart Energy Systems, or the course, visit the PJ SES course’s page or contact the AC Energy teaching responsible person Ogün Yurdakul.
Topics
Autonomous Distributed Energy Systems
Whereas in the traditional energy system, the most important decisions for ensuring a reliable supply were made manually in central control centers, the decentralization of the energy system leads to increasingly autonomous behavior at all levels.
More and more prosumers interact directly with other prosumers, aggregators, or local market platforms to optimize the energy supply, provide flexibility, and sell excess renewable energy. Active distribution grids monitor and predict their state also at the low-voltage level to intervene if needed.
These developments pose new research challenges. AI algorithms’ decisions must be reliable and even explainable for humans to enable the transition to trusted automated decisions. Cybersecurity must be ensured, also in a highly interconnected system.
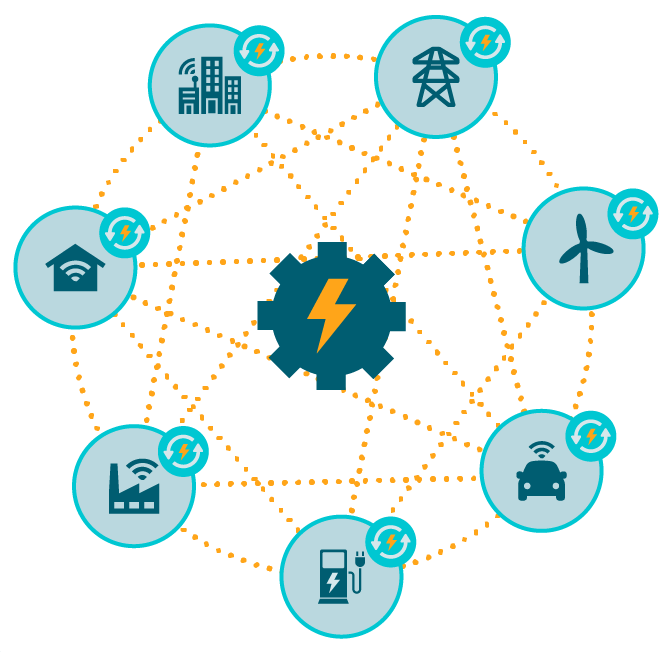
Energy Data Analytics
With decentralization and digitization of the energy system, more sensors are installed and vast amounts of data are collected for improving system operation and planning.
The data can be utilized to create digital twins of components in the system for detailed simulations to enable better decision support. Machine learning and data-driven modeling enable smarter predictive energy management by modeling uncertainties of renewable production and load behavior on all system levels. But sensors are not needed everywhere: Machine learning can be used to identify individual components in aggregated signals through non-intrusive load monitoring (NILM), can estimate states of unobserved parts of the grid, or synthesize realistic data, if no measured data is available. Data can also be used to predict different component’s failures by learning normal behavior and detect systematic deviations before the worst-case of an expensive failure happens.
Next to handling the vast volume, and ensuring the data’s quality, assuring the privacy of sensitive data is a relevant research challenge.
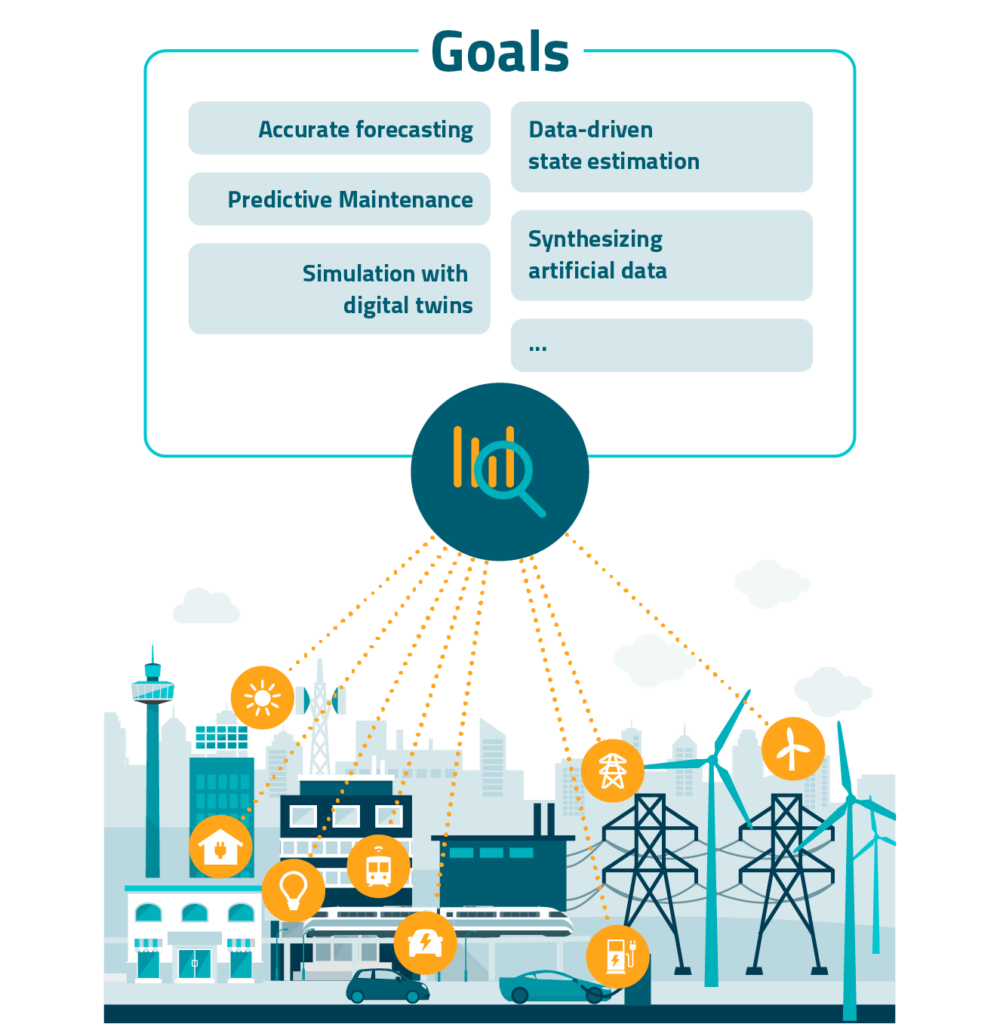
Future E-Mobility
With the mobility sector’s decarbonization, future mobility services will be tightly integrated with the energy system and the smart grid. At the same time, vehicles are becoming increasingly autonomous. The increasing autonomy poses new challenges to the integration with the energy system but offers great potential.
Electric vehicles that are connected to the grid can provide aggregated flexibility and energy storage services through controlled charging and vehicle-to-grid. Eventually, full-autonomous vehicles automatically charge and can move with grid capacity. But the transition to more service-oriented mobility influences the impact on the power grid. Automated charging influences the optimal planning of charging infrastructure.
The research challenges lay therefore in supporting the long-term planning of charging infrastructure and power grids, under uncertainty of how mobility patterns and autonomous driving technologies will develop.
