Autonomous Mobility Testbed
Motivation
The mobility of the future will be determined by the intelligent interplay of different autonomous mobility modalities. While the innovative power to achieve real autonomous mobility in industry continues to increase, the availability of pilot locations, where advanced levels of autonomy can be tested in detail, is a crucial cornerstone for achieving flawless autonomous vehicles and the associated services. With the Autonomous Driving Playground Testbed (ADP), the DAI laboratory offers an environment and platform to develop new skills and solutions, and for the validation and demonstration of future multimodal mobility concepts in which autonomous driving and the data and data intended for the implementation Service ecosystems are used.
Objective
The aim of the Autonomous Driving Playground is to provide data, platform, tools and infrastructure for
- the testing of AI algorithms, driving and networking functions of individual modalities in real operation,
- the testing of different modalities in their combination with each other, and
- the provision of historical and real-time mobility data and metrics for manufacturers, suppliers, developers and operators of mobility solutions.
The ADP Testbed is focused on testing bleeding edge autonomous driving solutions in a realistic environment, providing a rich, dense and urban setting in which any research involving advanced autonomy levels finds its challenges. This includes the physical test track, abundant sensory data obtained in vehicles and at the roadside from a wide range of sensor manufacturers, V2X communication, machine learning exploiting far and near edge computing infrastructure, intelligent Local Dynamic Map composition and exchange via V2X, and much more.
Challenges
The Interplay of sensors, IoT middlewares, cloud platforms and their AI-driven management of the underlying compute and machine learning components, the fusion of distributed sensory data, inter-operability of car and sensory equipment vendors, the V2X communication infrastructure based on flexible and programmable networks are some of the examples for challenges associated with our autonomous driving vision. The ADP Testbed should facilitate the research on autonomous driving with the overall aim of providing a playground for innovative solutions as well as making available a platform for testing matured industry-grade solutions.
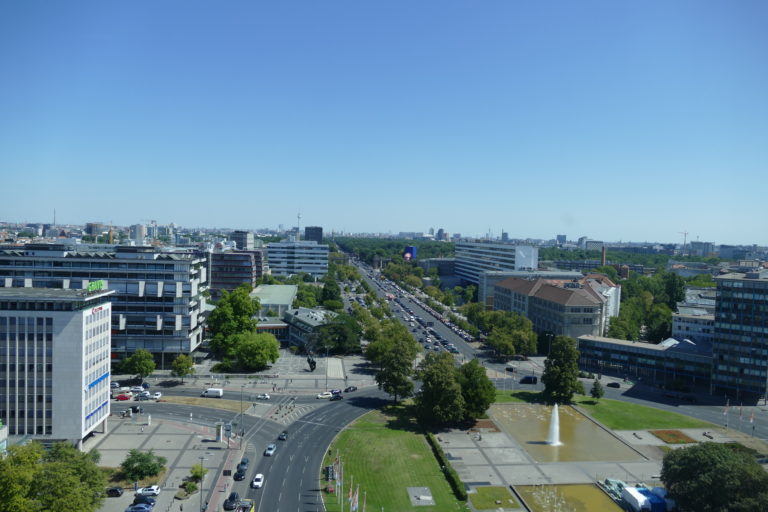
Test Field
The ADP Testbed is located on Straße des 17. Juni between Ernst-Reuter-Platz and the Brandenburg Gate. It is a nearly four-kilometer-long, busy federal road in the heart of Berlin with the following characteristics:
- Three lanes in both directions with a median
- Two large roundabouts, each with five entrances and exits
- 15 traffic lights
- More than 1,500 parking spaces (parallel and at an angle to the road, on the edge of the road and on the median and in separate parking areas)
- Bike lanes next to the road
- Crossing bike and footpaths
- Both wooded and built-up sections
- Bridges
- Occasional construction sites and road closures
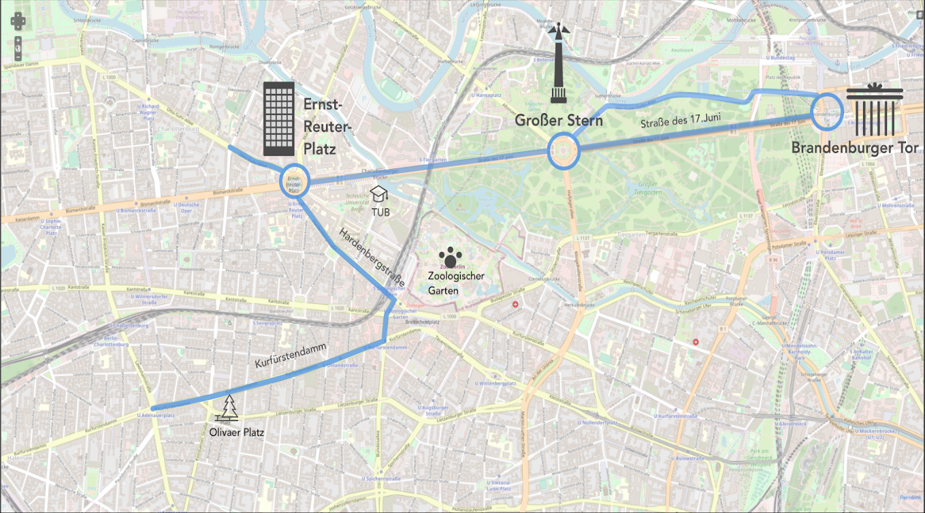
An extension of the test field to include John-Foster-Dulles-Allee, Otto-Suhr-Allee, Hardenbergstrasse and Kurfürstendamm is already planned in order to include both the parliamentary society and an important shopping mile.
Technology
The ADP Testbed consists of the following components which are under continuous development:
- Roadside sensors, for example, to record free parking spaces, road users, the condition of the road, the air quality and the weather conditions
- Edge computing infrastructure for local processing and analysis of data, creation of predictions and local decision making with low latency
- Cloud infrastructure for the global collection and analysis of all data, global decision-making and the operation of the control center
- Machine learning processes for the detection, classification and tracking of objects (trucks, cars, bicycles, pedestrians) based on traffic surveillance cameras
- Data analytics engine for analyzing sensor data and predicting future situations in order to be able to make the right decisions as early as possible
- V2X infrastructure both for warning of dangerous situations and for the transmission of current and future traffic light phases, aggregated sensor data, vehicle data and predictions
- IP-based communication infrastructure based on WLAN, microwave and 4G / 5G for synchronization between the cloud and the edge PCs or vehicles
- Test vehicle for testing new functions or different applications in a real environment
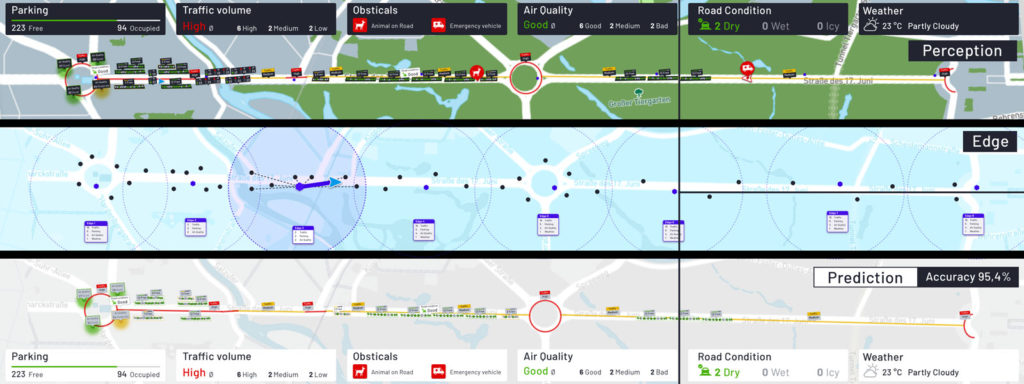
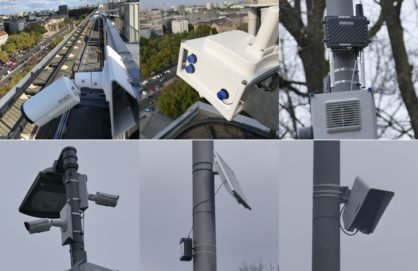
Test vehicle
A connected VW Tiguan is currently available as a test vehicle, which will soon be supplemented with automated driving functions.
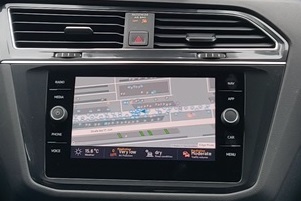
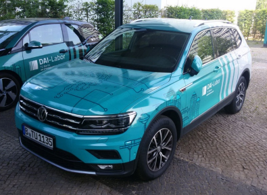
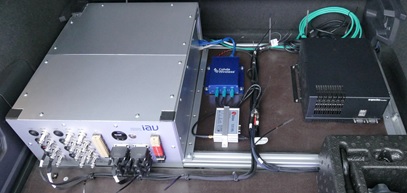
Other test vehicles such as an automated bus with 12 meters in length, an automated transporter and a delivery robot are already planned.
Our Offer
With the ADP we offer interested companies and institutions the following services (excerpt):
- Testing of new automated, connected and cooperative driving functions
- Sample applications and standard test cases are available
- Implementation of further digitization ideas, test cases or applications
Some examples of use are listed below:
- Integration of infrastructure and vehicle perception CPM, IVIM
- Infrastructure components (sensors, communication, computation) and applications
- Car-2-Car and Car-2-X communication based on the ITS G5 standard or custom
- Cooperative driving maneuvers, convoy (CAM, platooning)
- Dangerous situations (DENM)
- Traffic light information SPaT / MAP – GLOSA
- Parking, road conditions, weather, traffic conditions, air quality – perception and predictions
- Control center and traffic information for city administration or traffic control
- Scaling and distribution of the solution to edge, cloud and vehicle
- Provision of real-time data and predictions
- Use of data for all kinds of evaluations
- Definition of metrics for evaluation
Possible scenarios
Simple scenarios (ONE car, ego car):
- Speed: Top speed (50 km/h), adjusted speed (weather, recommended speed, GLOSA, traffic volume)
- Distance: Vehicle in front, side distance (moving and parked vehicles, cyclists, people)
- Keeping in lane, changing lanes, overtaking (non-cooperative, mixed traffic)
- Parking in and out (non-cooperative, mixed traffic)
- Roundabout
- Blind spot
- Emergency driving maneuvers: emergency braking, evasion
Connected scenarios (standards): Vehicle2Vehicle, Vehicle2Infrastructure, Infrastructure2Vehicle
- CAM: position, direction, speed
- DENM: Hazard messages, construction site, dynamic: pedestrians, cyclists, emergency braking
- SPaT / MAP: traffic light phases, intersection topology
- GLOSA: Green Wave Assistant, speed reduction
Connected scenarios (not (yet) standard): Cooperative perception:
- CPM / IVIM: Collective Perception Message (greater latency than CAM), Infrastructure to Vehicle Information Message (e.g. variable speed limit, construction sites)
- Further messages from Edge / Cloud for perception and predictions: weather, air quality, traffic situation, object detection (vehicles, bicycles, pedestrians)
Cooperative driving situations:
- Convoy / platooning based on V2V communication
- Stop & Go
- Cooperative parking in and out
- Cooperative lane change (in case of construction sites, lane blocked)
- Threading (zipper principle)
- Turn
Bad weather:
- (very) slippery surface
- Heavy rain, snow, fog
Relapse Scenarios:
- Driver takes over the driving task
- Risk-minimizing maneuvers (driver does not react)
Remote support or control (teleoperation) – different levels:
- High-level commands
- Assisting level
- Direct control