Energy Data Analytics
With decentralization and digitization of the energy system, more sensors are installed and vast amounts of data are collected for improving system operation and planning.
The data can be utilized to create digital twins of components in the system for detailed simulations to enable better decision support. Machine learning and data-driven modeling enable smarter predictive energy management by modeling uncertainties of renewable production and load behavior on all system levels. But sensors are not needed everywhere: Machine learning can be used to identify individual components in aggregated signals through non-intrusive load monitoring (NILM), can estimate states of unobserved parts of the grid, or synthesize realistic data, if no measured data is available. Data can also be used to predict different component’s failures by learning normal behavior and detect systematic deviations before the worst-case of an expensive failure happens.
Next to handling the vast volume, and ensuring the data’s quality, assuring the privacy of sensitive data is a relevant research challenge.
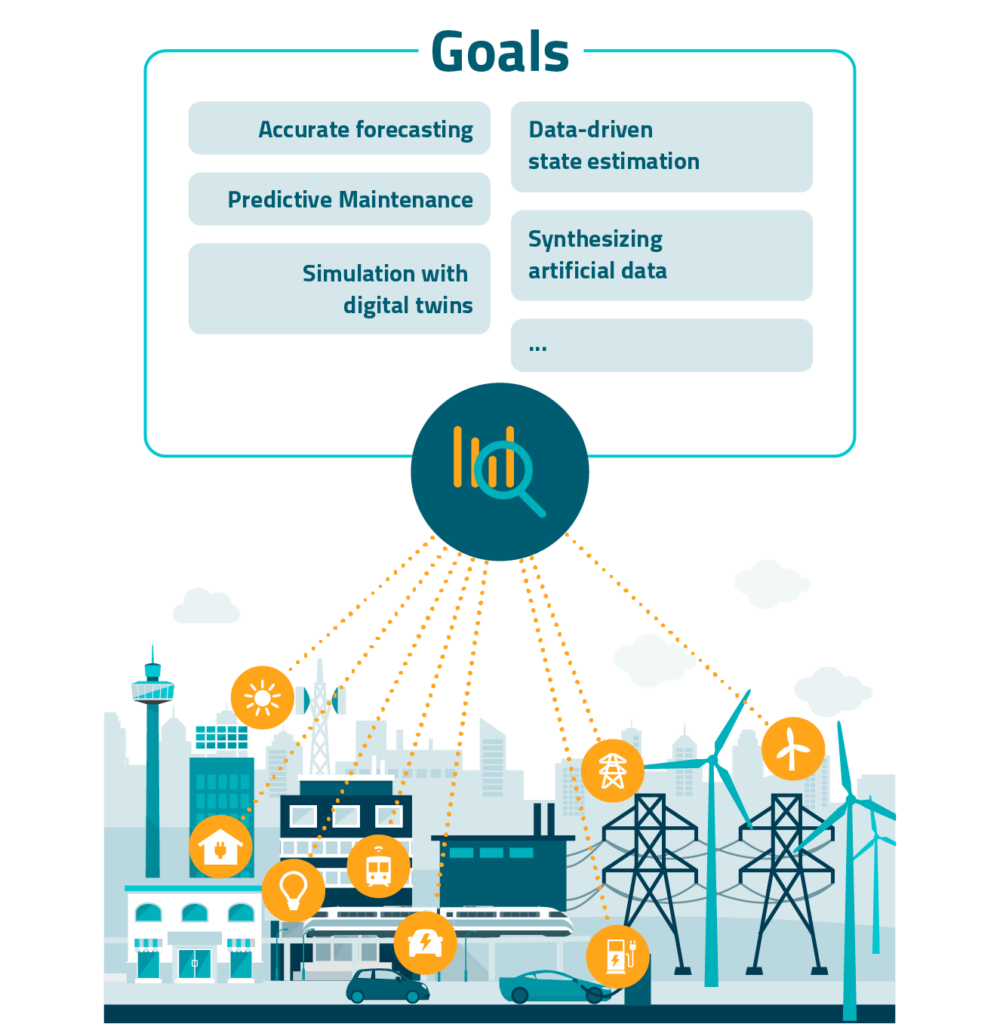